Assessing the effectiveness of static heuristics for scheduling lumber orders in the sawmilling production process
DOI:
https://doi.org/10.22320/s0718221x/2024.39Keywords:
Lumber planning, lumber scheduling, backorders cost, static heuristics, minimum production costs, sawmillsAbstract
Although optimization models can be used to plan the production process, in most cases static heuristics, such as earliest due date (E), longest processing time (L), and shortest processing time (S), are used because of their simplicity. This study aims to analyze the production cost of the static heuristics and to determine how this cost relates to the size of the production orders in the sawmilling industry. We set a planning problem with different orders and due dates and solved it using two cost-minimization models to compare their solutions. The first was a planning model (PL) where orders were split up into products demand by period, and the second, a planning scheduling (PS) where the sequence of processing orders based on static heuristics was assumed as known. In the latter, the minimum production cost for each static heuristic was found. In both models, the same resource constraints were assumed. The costs showed no significant changes based on order sizes. However, 0,5 % of orders were delayed using PS-E, and 17 % of orders were delayed using PL. PL was an efficient solution method when changing the orders´ size and when looking for the best static heuristic to process the orders. However, PS-E showed the ability to reduce the backlog close to zero while the PL backlog ratio was 17 %. No penalties were applied to backlogs due to their subjective nature; however, when shortages occurred, the demand was unmet or backlogged with substantial costs. Thus, in case the proposed method is adopted using a conservative backlog cost, a sawmill producing under the cut-to-order environment that produces 300000 m3 /year would reduce backlogged orders by 51000 m3. If the holding lumber cost is 2 $/m3, annual savings would be $408000.
Downloads
References
Ben Ali, M.; D’amours, S.; Gaudreault, J.; Carle, M.A. 2019. Integrating revenue management and sales and operations planning in a Make To Stock environment: softwood lumber case study. INFOR: Information Systems and Operation Research 57(2): 314-341. https://doi.org/10.1080/03155986.2018.1554420
Broz, D.; Vanzetti, N.; Corsano, G., Montagna, J.M. 2019. Goal programming application for the decision support in the daily production planning of sawmills. Forest Policy and Economics 102: 29-40. https://doi.org/10.1016/j.forpol.2019.02.004
Clark, A.R. 2003. Optimization approximations for capacity constrained material requirements planning. International Journal of Production Economics 84(2): 115-131. https://doi.org/10.1016/S0925-5273(02)00400-0
de Araujo, S.A.; Arenales, M.N.; Clark, A.R. 2007. Joint rolling horizon scheduling of materials processing and lot sizing with sequence dependent setups. Journal of Heuristics 13(4): 337-358. https://doi.org/10.1007/s10732-007-9011-9
Dumetz, L.; Gaudreault, J.; El-Haouzi, H.B.; Thomas, A.; Lehoux, N.; Marier, P. 2019. Simulation and Evaluation of Coordination Mechanisms for a Decentralized Lumber Production System with Coproduction. Forest Products Journal 69(4): 260-271. https://doi.org/10.13073/FPJ-D-19-00002
Gaudreault, J.; Frayret, J.M.; Rousseau, A.; D’Amours, S. 2011. Combined planning and scheduling in a divergent production system with coproduction: A case study in the lumber industry. Computers & Operations Research 38(9): 1238-1250. https://doi.org/10.1016/j.cor.2010.10.013
Gupta, S.; Starr, M. 2014. Production and Operations Management Systems. CRC Press: Boca Raton, The United States of America. https://doi.org/10.1201/b16470
Huka, A.M.; Gronalt, D. 2017. Model development and comparison of different heuristics for production planning in large volume softwood sawmills. Engineering Optimization 11(9): 1829-1847. http://dx.doi.org/10.1080/0305215X.2016.1271882
Kaltenbrunner, M.; Huka, M.A.; Gronalt, M. 2020. Adaptive Model Building Framework for Production Planning in the Primary Wood Industry. Forests 11(12): e1256. https://doi.org/10.3390/f11121256
Maccarthy, B.L.; Liu, J. 1993. Addressing the gap in scheduling research: a review of optimization and heuristic methods in production scheduling. International Journal of Production Research 31(1): 59-79. https://doi.org/10.1080/00207549308956713
Maness, T.C.; Adams, D.M. 1991. The combined optimization of log bucking and sawing strategies. Wood and Fiber Science 23(2): 296-314. https://wfs.swst.org/index.php/wfs/article/view/189
Maness, T.C.; Norton, S.E. 2002. Multiple Period Combined Optimization Approach to Forest Production Planning. Scandinavian Journal of Forest Research 17(5): 460-471. https://doi.org/10.1080/028275802320435478
Marier, P.; Gaudreault, J.; Robichaud, B. 2014. Implementing a MIP model to Plan and Schedule Wood Finishing Operations in a Sawmill: Lessons Learned. In Proceedings of the 10th International Conference of Modeling and Simulation “Toward Circular Economy”: Nancy, France. 5-7 November 2014, pp.1-8. https://hal.science/hal-01166659/
Maturana, S.; Pizani, E.; Vera, J. 2010. Scheduling production for a sawmill: A comparison of a mathematical model versus a heuristic. Computers and Industrial Engineering 59(4): 667-674. https://doi.org/10.1016/j.cie.2010.07.016
Pradenas, L.; Peñailillo, F.; Ferland, J. 2004. Aggregate Production Planning Problem, A new Algorithm. Electronic Notes in Discrete Mathematics 18: 193-199. https://doi.org/10.1016/j.endm.2004.06.031
Possani, E. 2001. Lot Streaming and Batch Scheduling: splitting and grouping jobs to improve production efficiency. Doctoral Thesis. University of Southampton. 162p. http://eprints.soton.ac.uk/id/eprint/50621
Singer, M.; Donoso, P. 2007. Internal supply chain management in the Chilean sawmill industry. International Journal of Operations & Production Management 27(5): 524-541. https://doi.org/10.1108/01443570710742393
Sipper, D.; Bulfin, R.L. 1997. Production: planning, control, and integration. McGraw-Hill International Ed., New York, The United States of America. https://cir.nii.ac.jp/crid/1130282270676821760
Todoroki, C.; Rönnqvist, M. 2010. Dynamic Control of Timber Production at a Sawmill with Log Sawing Optimization. Scandinavian Journal of Forest Research 17(1): 79-89. https://doi.org/10.1080/028275802317221118
Vanzetti, N.; Broz, D.; Corsano, G.; Montagna, J.M. 2019. A detailed mathematical programming model for the optimal daily planning of sawmills. Canadian Journal of Forest Research 49(11): 1400-1411. https://doi.org/10.1139/cjfr-2019-0144
Vanzetti, N.; Broz, D.; Corsano, G.; Montagna, J.M. 2018. An optimization approach for multiperiod production planning in a sawmill. Forest Policy and Economics 97: 1-8. https://doi.org/10.1016/j.for-pol.2018.09.001
Vergara, F.P.; Palma, C.D.; Sepulveda, H. 2015. A comparison of optimization models for lumber production planning. Bosque 36(2): 239-246. http://dx.doi.org/10.4067/S0717-92002015000200009
Wery, J.; Marier, P., Gaudreault, J.; Thomas, A. 2014. Decision-making framework for tactical planning taking into account market opportunities (new products and new suppliers) in a coproduction context. In Proceedings of the 10th International Conference of Modeling and Simulation MOSIM’14: Nancy, France. 5-7 November 2014, pp.2-10 https://hal.science/hal-01094799
West, K.D. 1989. Order Backlogs and Production Smoothing. The Rational Expectations Equilibrium Inventory Model: Theory and Applications. 1st Edition. Tryphon Kollintzas Editorial: Amsterdam, Nederland. https://doi.org/10.1007/978-1-4684-6374-3_6
Yaghubian, A.R.; Hodgson, T.J.; Joines, J.A. 2001. Dry or buy decision support for dry kiln scheduling in furniture production. IIE Transactions 33(2): 131-136. https://doi.org/10.1080/07408170108936814
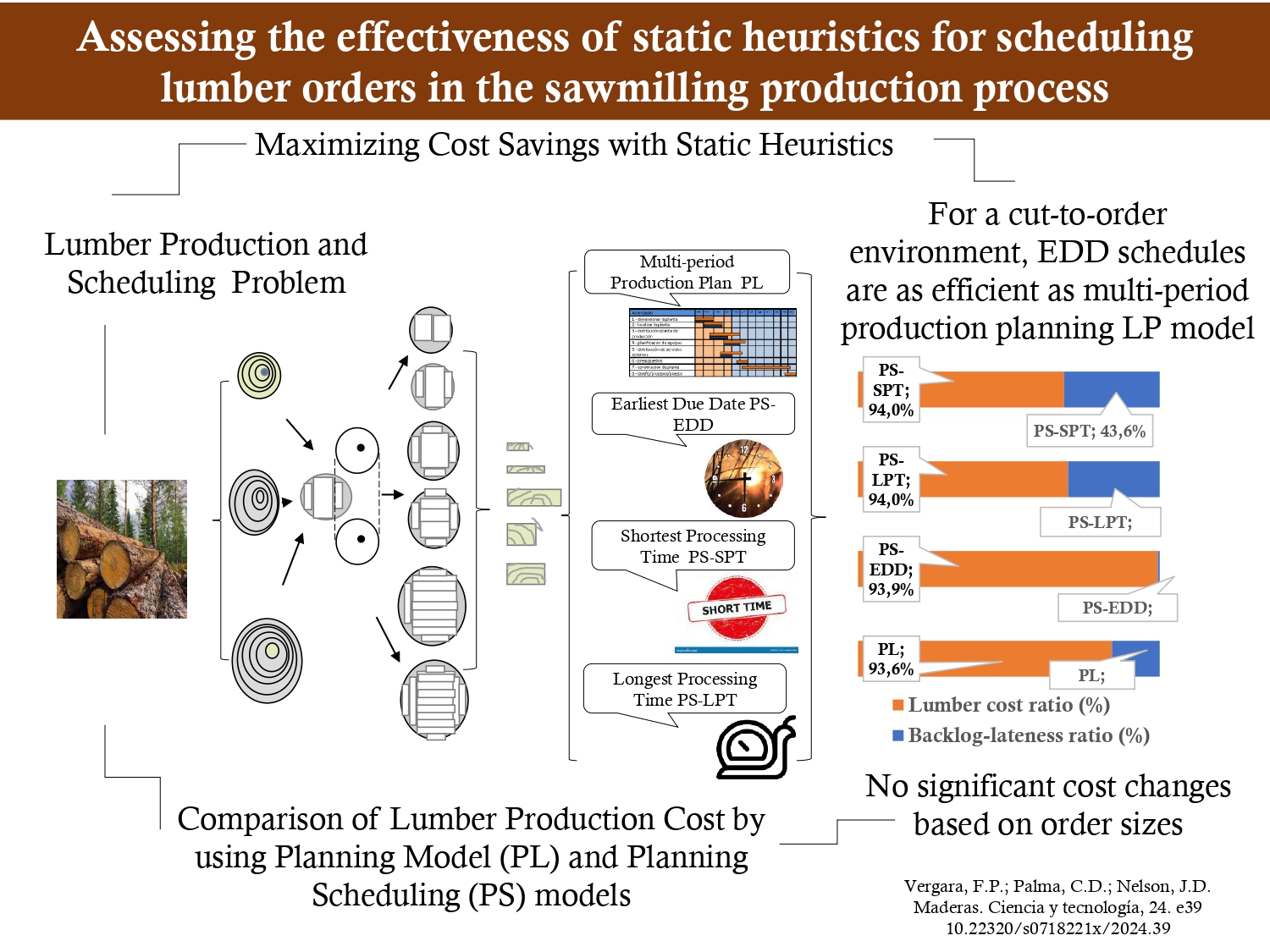
Downloads
Published
How to Cite
Issue
Section
License

This work is licensed under a Creative Commons Attribution 4.0 International License.
Los autores/as conservarán sus derechos de autor y garantizarán a la revista el derecho de primera publicación de su obra, el cuál estará simultáneamente sujeto a la Licencia de Reconocimiento de Creative Commons CC-BY que permite a terceros compartir la obra siempre que se indique su autor y su primera publicación esta revista.